Comprehensive AI Integration in DevOps: Enhancing Automation and Optimization
Solusian
Published on Apr 07, 2025
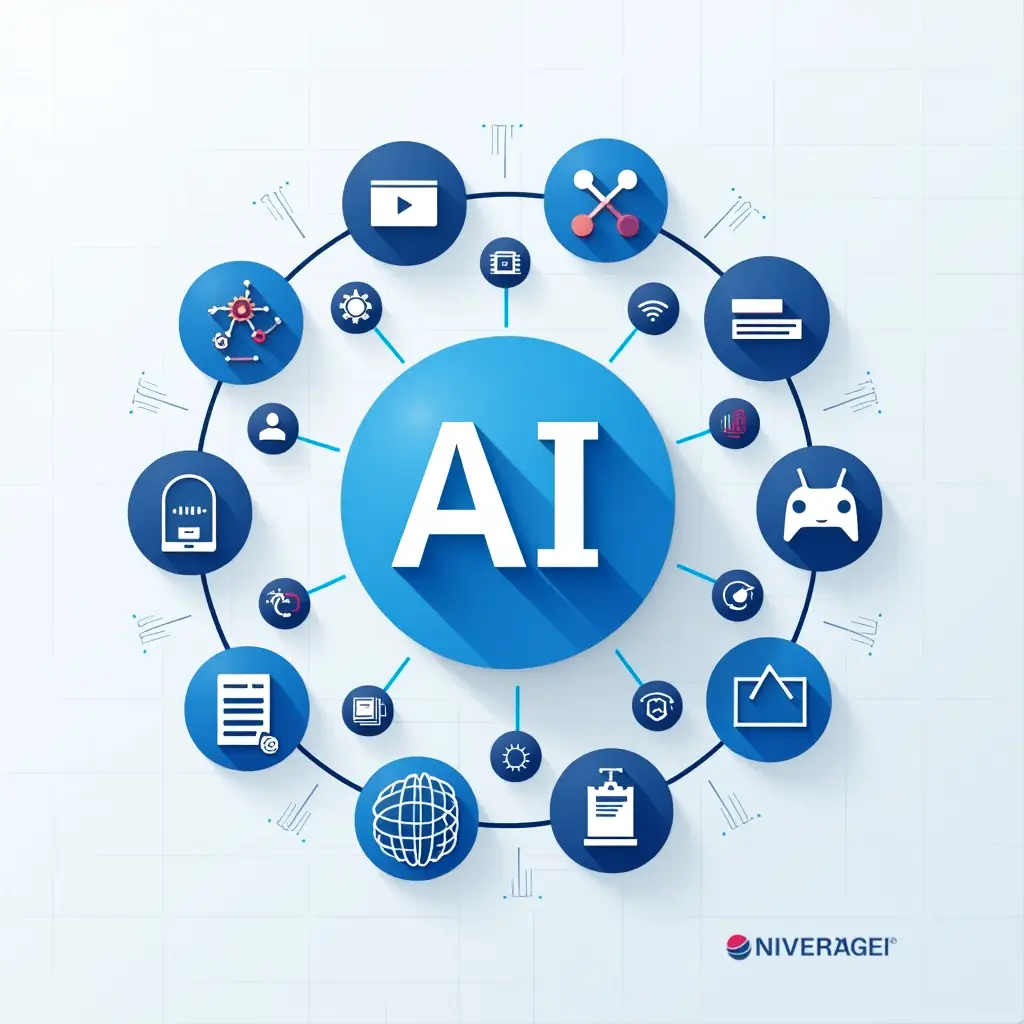
The integration of Artificial Intelligence (AI) into DevOps practices represents one of the most significant technological evolutions in software development and IT operations. As organizations strive for faster deployment cycles, improved code quality, and more reliable systems, AI offers powerful capabilities to automate, optimize, and enhance the entire DevOps pipeline.
Understanding AI in DevOps: The Foundation
At its core, AI in DevOps aims to automate repetitive tasks, provide intelligent insights, and optimize workflows across the software development lifecycle. Before diving into specific implementations, let’s understand the key areas where AI adds value:
Areas of AI Application in DevOps
- Automated Testing and Quality Assurance: AI can generate test cases, predict test coverage, identify regression risks, and prioritize tests based on code changes.
- Predictive Analytics: AI algorithms can analyze historical data to predict potential deployment failures, performance bottlenecks, or security vulnerabilities.
- Intelligent Monitoring: AI-based monitoring tools can detect anomalies, identify root causes, and even suggest remediation steps automatically.
- Code Quality and Review: AI can analyze code patterns, identify potential bugs, and suggest improvements before code is merged.
- Release Management: AI can optimize release schedules, predict deployment risks, and recommend optimal deployment strategies.
Getting Started with AI-DevOps Integration
Step 1: Assess Your DevOps Maturity
Before integrating AI, it’s important to understand your current DevOps maturity level:
- Does your organization have a well-defined CI/CD pipeline?
- Are development, testing, and operations teams collaborating effectively?
- Do you have monitoring and logging systems in place?
- How are you currently handling infrastructure provisioning?
Step 2: Identify Integration Points
Identify specific points in your DevOps workflow where AI can add immediate value:
- Development Phase: Code quality analysis, security scanning
- Testing Phase: Test generation, test optimization
- Deployment Phase: Deployment risk analysis, blue-green deployment decisions
- Monitoring Phase: Anomaly detection, predictive maintenance
Step 3: Select the Right Tools
Several AI-powered tools can enhance your DevOps pipeline:
- Code Analysis: DeepCode, CodeGuru, Snyk
- Testing: Mabl, Testim, Applitools
- Deployment: Harness AI, OpsMx
- Monitoring: Dynatrace, DataDog, New Relic
Enabling AI Debugging in DevOps
Debugging is often one of the most time-consuming aspects of software development. AI-powered debugging tools can significantly reduce this time by automatically identifying issues and suggesting fixes.
Implementing AI Debugging
- Set Up Comprehensive Logging
AI debugging systems rely heavily on quality data. Implement structured logging across your application:
python1# Example of structured logging in Python2import logging3import json45def structured_log(event_type, data):6 log_entry = {7 "event_type": event_type,8 "data": data,9 "timestamp": datetime.now().isoformat()10 }11 logging.info(json.dumps(log_entry))
- Integrate AI Debugging Tools
Several tools offer AI-powered debugging capabilities:
- Cursor: An AI-powered code editor with debugging capabilities
- DeepCode: Uses machine learning to find bugs and suggest fixes
- Microsoft’s IntelliCode: Provides AI-assisted code completions and bug detection
- Train Models on Your Codebase
For more advanced implementations, consider training custom models on your specific codebase:
python1# Example using a simple ML model to identify bug patterns2from sklearn.ensemble import RandomForestClassifier34# Train on historical bug data5model = RandomForestClassifier()6model.fit(historical_code_features, bug_labels)78# Use for prediction9def predict_bugs(code_features):10 return model.predict(code_features)
- Implement Automated Root Cause Analysis
AI can help identify the root cause of production issues:
plaintext1# Example configuration for an AI-based RCA tool2analysis:3 data_sources:4 - logs5 - metrics6 - traces7 correlation_threshold: 0.758 learning_mode: active9 notification:10 channels:11 - slack12 - email
Integrating AI with Jenkins
Jenkins remains one of the most popular CI/CD tools, and integrating AI capabilities can significantly enhance its functionality.
Setting Up Jenkins with AI Capabilities
- Install Required Plugins
Several Jenkins plugins provide AI capabilities:
- Jenkins AI Plugin: Enables AI-powered insights into your builds
- Code Quality Analyzer: Integrates with AI-powered code analysis tools
- ML Prediction Plugin: Predicts build success/failure
- Configure Your Jenkinsfile
Here’s an example of a Jenkinsfile that incorporates AI-powered testing and code analysis:
plaintext1pipeline {2 agent any34 stages {5 stage('Build') {6 steps {7 sh 'mvn -B -DskipTests clean package'8 }9 }1011 stage('AI Code Analysis') {12 steps {13 // Integrate with AI code analysis tool14 sh 'ai-code-scan --repo-path .'1516 // Process results17 script {18 def aiResults = readJSON file: 'ai-scan-results.json'19 if (aiResults.critical_issues > 0) {20 error "Critical issues found in code scan"21 }22 }23 }24 }2526 stage('AI Test Optimization') {27 steps {28 // Use AI to determine which tests to run29 script {30 def testsToRun = sh(script: 'ai-test-optimizer --changes-only', returnStdout: true).trim()31 if (testsToRun) {32 sh "mvn -B test -Dtests=${testsToRun}"33 } else {34 sh "mvn -B test"35 }36 }37 }38 }3940 stage('Deploy') {41 steps {42 // AI-driven deployment decision43 script {44 def deploymentRisk = sh(script: 'ai-deployment-risk-analyzer', returnStdout: true).trim()45 if (deploymentRisk == 'LOW') {46 sh 'deploy-to-production'47 } else {48 sh 'deploy-to-staging'49 // Notify for manual approval50 notifyDevTeam("High deployment risk detected. Manual approval required.")51 }52 }53 }54 }55 }56}
- Implement AI-Powered Build Optimization
You can optimize your Jenkins builds using AI predictions:
plaintext1// In your Jenkinsfile2stage('Build Optimization') {3 steps {4 script {5 // Get AI recommendation for optimal build configuration6 def optimizedConfig = sh(script: 'ai-build-optimizer --analyze-history', returnStdout: true).trim()78 // Apply the optimized configuration9 withEnv(["BUILD_CONFIG=${optimizedConfig}"]) {10 sh 'mvn -B -DskipTests clean package'11 }12 }13 }14}
- Predictive Testing with Jenkins
Implement predictive test selection to run only the tests that are likely to fail:
plaintext1stage('Predictive Testing') {2 steps {3 script {4 // Get AI prediction on which tests might fail5 def testPredictions = readJSON(text: sh(script: 'ai-test-predictor', returnStdout: true))67 // Run tests with high failure probability first8 def highRiskTests = testPredictions.highRiskTests.join(',')9 if (highRiskTests) {10 sh "mvn test -Dtest=${highRiskTests}"11 }1213 // Run remaining tests14 sh "mvn test -Dtest=${testPredictions.remainingTests.join(',')}"15 }16 }17}
Real-World Implementation Strategies
1. Start Small with Focused AI Integration
Rather than implementing AI across your entire DevOps pipeline at once, begin with a specific area where AI can provide immediate value:
plaintext1# Example: Starting with AI-powered code reviews2ai-code-review:3 enabled: true4 repositories:5 - main-service6 - auth-service7 focus_areas:8 - security9 - performance10 notification:11 slack_channel: "#code-reviews"
2. Implement AI-Powered Incident Management
Use AI to detect anomalies and predict potential incidents:
plaintext1# AI incident management configuration2incident_prediction:3 data_sources:4 - system_metrics5 - application_logs6 - previous_incidents7 alert_threshold: 0.75 # Probability threshold for alerting8 auto_remediation:9 enabled: true10 allowed_actions:11 - restart_service12 - scale_up13 - rollback_deployment
3. Leverage AI for Infrastructure Optimization
AI can help optimize your cloud resources and infrastructure:
plaintext1# AI infrastructure optimizer2infrastructure_optimization:3 target_resources:4 - kubernetes_clusters5 - database_instances6 - load_balancers7 optimization_goals:8 - cost_reduction9 - performance_improvement10 implementation:11 - recommendation_only: true # Start with recommendations before automated changes
Best Practices for AI in DevOps
To ensure successful AI integration with your DevOps processes:
- Ensure Data Quality: AI systems are only as good as the data they learn from. Implement comprehensive logging and monitoring.
- Start with Augmentation, Not Replacement: Use AI to augment human decision-making before fully automating processes.
- Maintain Explainability: Choose AI tools that provide explanations for their recommendations or decisions.
- Continuous Learning: Set up feedback loops to improve your AI models over time.
- Security First: Ensure AI components don’t introduce new security vulnerabilities.
- Measure Impact: Define clear metrics to measure the impact of AI on your DevOps processes.
Common Challenges and Solutions
Challenge 1: Resistance to Change
Solution: Start with low-risk areas, demonstrate value, and involve the team in the selection and implementation of AI tools.
Challenge 2: Data Quality Issues
Solution: Implement data validation, cleaning, and governance processes before feeding data to AI systems.
Challenge 3: Integration Complexity
Solution: Use APIs and standardized interfaces between AI tools and your existing DevOps toolchain.
Challenge 4: Lack of Expertise
Solution: Invest in training or consider partnerships with AI experts or consultants.
Integrating AI into DevOps represents a significant opportunity to enhance development speed, quality, and reliability. By starting with specific use cases, choosing the right tools, and following best practices, organizations can gradually build an AI-enhanced DevOps pipeline that delivers better software faster.
Remember that AI in DevOps is not about replacing human expertise but augmenting it. The most successful implementations combine the strengths of human creativity and judgment with AI’s ability to process vast amounts of data and identify patterns.
As AI technologies continue to evolve, stay informed about new capabilities and tools that can further enhance your DevOps processes. The journey toward AI-powered DevOps is ongoing, with continuous improvements and optimizations along the way.
FAQs
1. What are the minimum requirements for implementing AI in a DevOps pipeline?
To implement AI in your DevOps pipeline, you need a well-established CI/CD process, comprehensive logging and monitoring systems, and sufficient historical data (at least a few months of deployment, testing, and incident data). You’ll also need either in-house data science expertise or access to pre-built AI tools specifically designed for DevOps use cases.
2. How can I measure the ROI of AI implementation in DevOps?
Measure the ROI by tracking metrics before and after AI implementation: deployment frequency, lead time for changes, change failure rate, and mean time to recovery (MTTR). Additionally, track specific metrics related to your implementation goals, such as reduction in testing time, decrease in production incidents, or improvements in code quality scores.
3. Is it possible to implement AI in DevOps for small teams with limited resources?
Yes, small teams can implement AI in DevOps by starting with pre-built, SaaS-based AI tools that require minimal setup and maintenance. Focus on one specific challenge (such as test optimization or code quality) rather than trying to implement AI across the entire pipeline. Many vendors offer free tiers or startup pricing that makes these tools accessible to smaller teams.