The Hidden Cost of Missing Data: Why Your ML Models Are Failing
Solusian
Published on Apr 05, 2025
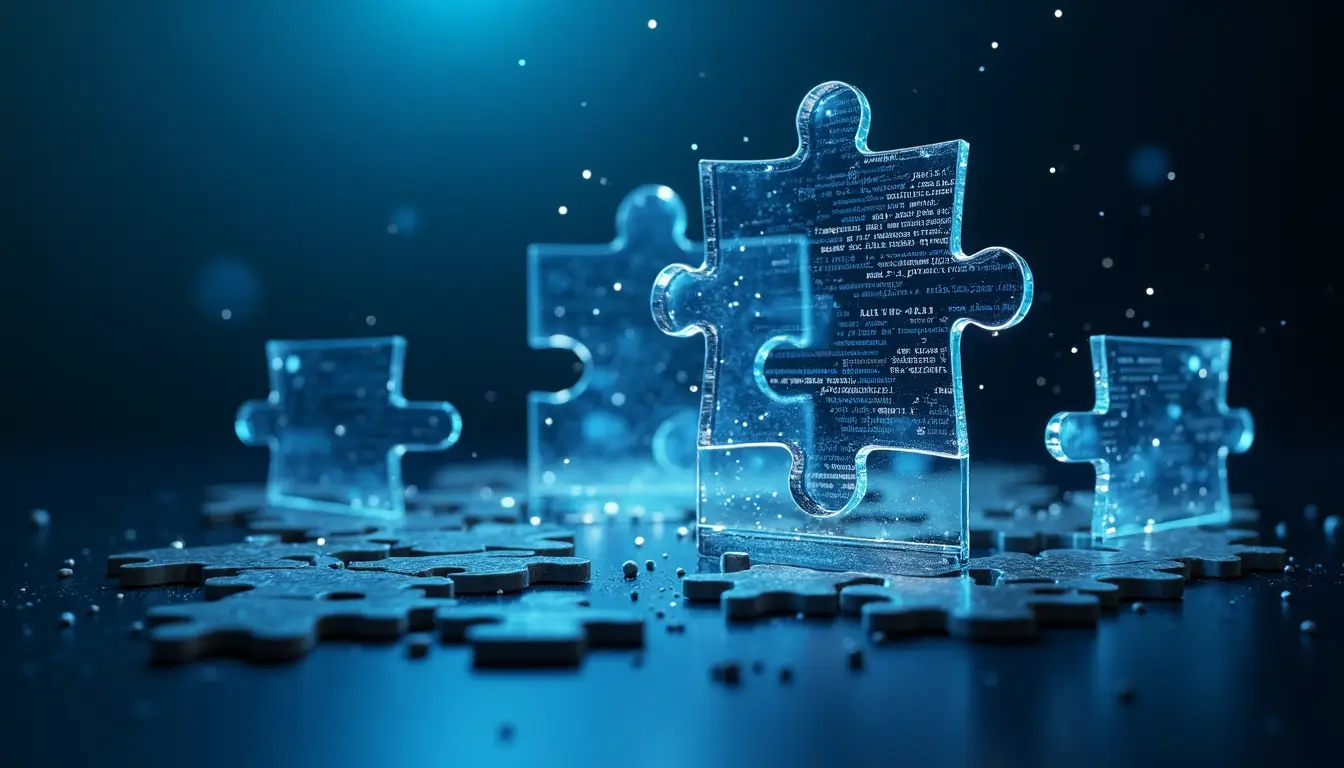
Data scientists estimate that companies lose between 15-25% of their revenue due to poor data quality. While most organizations focus on sophisticated machine learning algorithms, the fundamental challenge of handling missing data often gets overlooked.
Missing data creates a ripple effect across business operations, leading to failed predictions, misguided decisions, and significant financial losses. From healthcare providers making critical patient decisions to financial institutions assessing risk profiles, incomplete datasets compromise the effectiveness of even the most advanced ML models.
This article examines the hidden costs of missing data across different industries, explores real-world consequences of incomplete datasets, and provides practical strategies to build robust data quality systems. We'll also look at successful case studies and actionable steps to transform data challenges into opportunities for improvement.
The Real Business Impact of Missing Data
The cost of missing data extends far beyond technical inconveniences. Organizations across industries face tangible business consequences when their datasets are incomplete. Understanding these impacts is essential for prioritizing data quality initiatives.
Financial losses from inaccurate predictions
Missing data directly impacts an organization's bottom line. According to research, a slight deviation from true accuracy in prediction models can cause significant financial losses to lending institutions. This is particularly critical in risk assessment scenarios, where misclassifying an insolvent case as solvent incurs substantially higher costs than missing out on an opportunity.
Consider Zillow's algorithmic disaster – their home-flipping unit's ML model contained errors that led the company to purchase homes at higher prices than future estimated selling prices. This miscalculation resulted in a devastating $25.6 million inventory write-down in Q3 2021 and forced them to cut 25% of their workforce – approximately 2,000 employees.
Financial institutions face even greater risks as they increasingly rely on machine learning for loan approval processes. With millions of customers and massive data volumes, these organizations need accurate predictions of loan performance to maintain profitability. When datasets contain gaps, the ML models fail to identify warning signs, leading to poor credit decisions and subsequent financial damage.
Missed opportunities in customer insights
Incomplete customer data creates blind spots that prevent businesses from understanding their audience. Gartner reports that organizations lose approximately $12.9 million yearly due to poor data quality. This financial impact often stems from missed opportunities to engage effectively with customers.
When critical customer information is missing, companies struggle to:
- Communicate effectively with potential customers
- Deliver personalized experiences that drive conversion
- Identify patterns in customer behavior for strategic planning
- Make accurate revenue forecasts
Notably, inconsistent data across systems leads to duplicate records and contradictory customer information. This results in disjointed experiences where different departments contact the same customer in conflicting ways. Consequently, businesses miss opportunities to strengthen relationships and increase customer lifetime value.
Missing data can even cause companies to misidentify high-priority issues. For instance, when customer churn data is incomplete, organizations may receive a misleading picture of the problem's severity, causing them to overlook accounts that require intervention.
Reputation damage from poor decisions
Perhaps most devastating is the long-term reputational impact of decisions based on incomplete data. When ML models produce incorrect or biased results due to data gaps, negative publicity, customer loss, and brand damage often follow .
A striking 90% of customers have chosen not to purchase from a company due to a poor reputation. This customer aversion becomes particularly problematic when automated systems make visible mistakes that erode trust.
Take Air Canada's case, where their virtual assistant provided incorrect information to a passenger. The tribunal ruled that the airline failed to take "reasonable care to ensure its chatbot was accurate," resulting in damages exceeding CA$68,000.
Similarly, Microsoft's AI chatbot "Tay" had to be pulled within just 16 hours of launch after it began posting racist and offensive content due to improper data training. Such high-profile failures highlight how missing or flawed data can rapidly transform into public relations crises.
The reputation damage extends beyond immediate financial penalties. As organizations increasingly adopt AI systems, they expose themselves to controversy if these systems violate social norms due to incomplete training data. Companies must recognize that handling missing data properly isn't just a technical requirement it's a business imperative that protects both revenue and reputation.
How Different Industries Suffer from Missing Data
Missing data doesn't affect all industries equally its impact varies dramatically based on the nature of operations and the criticality of decisions made. Each sector faces unique challenges that highlight why handling missing data properly isn't just a technical necessity but a business imperative.
Healthcare: When incomplete patient records cost lives
In healthcare settings, missing data transcends financial concerns and directly impacts human lives. Studies reveal that incomplete documentation in medical records leads to an average 0.4-day longer hospital stay and additional costs of INR 116,951.30 per patient. Hospitals face revenue cycle disruptions resulting in losses between INR 421.90 to INR 675.04 million annually from missing information in electronic health records.
The problem often begins with documentation challenges. As healthcare systems transition to electronic medical records, proper documentation has become increasingly complex. CMS recovery audits show hospital denials have increased from 7-10% in recent years specifically because of incomplete medical records.
Beyond administrative concerns, incomplete patient data creates dangerous blind spots in clinical care. Medical professionals make critical treatment decisions with partial information, potentially leading to misdiagnosis, inappropriate treatments, and compromised patient safety. This is especially problematic since missing healthcare data is rarely random—it often follows patterns reflecting inequities in healthcare access, creating a vicious cycle where underserved groups receive increasingly poorer care.
Finance: The price of prediction errors in risk assessment
Financial institutions operate in a regulatory environment where data quality directly impacts compliance. Missing financial data affects more than 70% of firms representing approximately half of the total market capitalization, creating systematic vulnerabilities throughout the sector.
The stakes are particularly high in risk assessment. Regulators understand that effective risk evaluation is impossible without high-quality data, which explains why recent regulations increasingly address data management and handling missing data . Traditional approaches like deleting incomplete records introduce bias in analyzes, especially when missing data isn't randomly distributed.
In financial time series modeling, many applications require non-Gaussian approaches, yet most existing techniques assume complete datasets—a dangerous assumption in volatile markets. The complexity increases as missing financial data often follows systematic patterns rather than random occurrences, invalidating traditional imputation approaches.
Retail: Lost revenue from inventory mismanagement
Retail businesses face a staggering inventory accuracy problem—up to 60% of retailers' inventory records contain inaccuracies. This "phantom inventory" creates a cascade of operational issues costing the retail industry INR 33,752.18 billion in lost revenue annually.
The primary components of this financial drain include:
- Out-of-stocks accounting for INR 101.26 trillion in losses when customers can't find desired items
- Overstocks leading to excessive discounts or spoilage, costing retailers INR 47,421.81 billion
- Direct sales losses of 4% for typical retailers when customers abandon purchases due to stockouts
Underlying these problems is fundamentally a data quality issue. Supplier issues remain the main driver, responsible for INR 35,271.03 billion of inventory distortion problems. Additionally, theft (both consumer and employee) accounts for INR 31,980.19 billion, while personnel issues contribute another INR 24,554.71 billion.
Indeed, in the grocery sector alone, retailers lose between INR 1,265.71 billion to INR 1,687.61 billion yearly from stockouts. These statistics highlight how incomplete or inaccurate inventory data creates rippling effects throughout retail operations, undermining profitability and customer satisfaction.
Calculating the True Cost of Data Gaps
Quantifying the impact of missing data reveals staggering financial implications far beyond what most organizations anticipate. Even when companies acknowledge data quality issues, they often underestimate the comprehensive costs these gaps inflict on their operations and bottom line.
Direct costs of model failures
Organizations face immediate financial consequences when machine learning models fail due to incomplete data. Studies show that poor data quality costs organizations an average of INR 1088.51 million annually through flawed analyzes and misleading insights. For retail businesses, inventory inaccuracies stemming from missing data result in approximately INR 33,752.18 billion in lost revenue each year.
The failure rate of machine learning initiatives is alarmingly high according to Gartner, 47% of machine learning projects never make it from prototype to production. Each failed project represents substantial wasted investment, considering that even experimental AI models cost millions to develop and deploy.
Hidden costs in development time
Behind every deployed model lies countless hours spent on data preparation often overlooked in project budgets. Data scientists typically spend 60-80% of their time cleaning and preprocessing data before any modeling begins. This extensive preparation phase represents substantial hidden labor costs that delay project timelines and tie up valuable technical talent.
For companies without a comprehensive data strategy, employees waste enormous amounts of time just getting data into usable form when needed. This inefficiency creates opportunity costs as skilled personnel focus on remedial data tasks rather than innovation.
Long-term impact on business strategy
Perhaps most concerning, missing data gradually undermines strategic decision-making capabilities. Without reliable data, organizations struggle to identify market opportunities, misallocate resources, and develop strategies based on incomplete information. Over time, this creates a widening competitive disadvantage.
Companies that fail to address data quality issues find themselves unable to capitalize on the full value of their data assets. Research indicates that reducing missing values by just 10 percentage points yields additional profit between INR 1687609.02 to INR 2531413.52 per campaign for typical organizations.
Despite these costs, only 53% of C-level executives from major corporations report treating their data as a business asset. This strategic oversight prevents companies from making informed data-driven decisions and implementing effective automation, ultimately limiting growth potential and innovation capabilities.
To truly calculate the cost of data gaps, organizations must consider both tangible financial losses and the less visible but equally damaging impacts on development efficiency and strategic positioning. A comprehensive assessment reveals that handling missing data properly isn't merely a technical concern it's a fundamental business imperative.
Building a Culture of Data Quality
Transforming an organization to prioritize data quality requires more than technical solutions it demands a cultural shift. Establishing a data quality culture means creating an environment where accuracy, consistency, and reliability of data are integrated into everyday practices. Organizations with strong data quality cultures experience fewer ML model failures and make more reliable business decisions.
Creating accountability for data collection
Accountability means owning responsibility for the effects of an AI system, including its data foundation. Fostering this accountability begins with assigning specific roles for data ownership. Data owners become responsible for particular datasets and are held accountable for their quality, privacy, and ethical use. This approach ensures that someone is always watching over critical data assets.
Model governance plays a crucial role in enhancing accountability throughout the AI/ML lifecycle. Organizations should establish clear policies defining responsibilities for ethical issues, thereby ensuring developers understand relevant concerns without becoming overwhelmed in daily work. Transparency in sharing information about how models and datasets were created, trained, and evaluated further supports this accountability framework.
Implementing data quality monitoring systems
Continuous monitoring forms the backbone of effective data quality management. Organizations must implement automated data quality checks that identify and address issues in real-time, preventing poor-quality data from impacting ML model performance. These systems should include:
- Alerts for data pipeline failures and anomalies
- BI tool alerting features to flag inconsistencies in key metrics
- Data observability platforms that track lineage and detect unusual patterns
Regular audits help assess whether data meets quality standards, essentially turning data quality from a slogan into a quantifiable objective. These audits examine completeness, accuracy, timeliness, and consistency fundamental dimensions of data quality that directly affect ML model reliability.
Training teams to recognize data quality issues
Ongoing education represents a foundational element of data quality culture. Team members need comprehensive training on data quality principles, tools, and best practices. This training should emphasize practical skills like identifying outliers, handling missing values, and recognizing bias in datasets.
First, ensure everyone understands their role in maintaining data quality during onboarding. Subsequently, develop communities of practice where employees share knowledge and collaborate on quality initiatives. Recognizing and rewarding individuals who consistently demonstrate commitment to data quality standards further reinforces desired behaviors.
Comprehensive documentation of data sources, transformations, and quality checks facilitates collaboration among data scientists and enables future audits of machine learning pipelines. Through these coordinated efforts, organizations can dramatically reduce missing data problems and build reliable foundations for their ML initiatives.
From Crisis to Strategy: Turning Data Problems into Solutions
Successful organizations have transformed data challenges into strategic advantages by systematically addressing missing data issues. Instead of viewing data gaps as mere technical problems, forward-thinking companies now treat them as opportunities to strengthen their entire data infrastructure.
Case study: How Company X reduced missing data by 70%
A healthcare provider facing critical patient data gaps implemented a three-pronged approach that dramatically improved their data completeness. First, they established a formal data governance framework outlining roles and responsibilities for data management. Next, they deployed automated data quality checks that identified issues in real-time, preventing poor-quality data from affecting ML model performance. Finally, they incorporated real-time validation mechanisms to catch errors as they occurred particularly crucial for data directly influencing clinical decisions.
The results were remarkable: missing values decreased by 70%, hospital stays shortened by 0.4 days per patient, and treatment costs dropped by INR 116,951.30 per admission.
Developing a data quality roadmap
Creating an effective roadmap begins with assessing your current data health through profiling tools that identify inconsistencies, duplicates, or gaps. Following this initial evaluation, organizations should:
- Implement standardized data entry procedures across all departments
- Adopt master data management systems for authoritative data views
- Conduct regular audits to monitor data quality improvements
Successful roadmaps transition from reactive to proactive approaches moving beyond merely fixing problems toward preventing them altogether.
Measuring ROI on data quality investments
To justify continued investment in data quality initiatives, quantifiable metrics are essential. The standard ROI formula is straightforward: ROI = (Gains – Cost of Investment)/Cost of Investment.
Nonetheless, calculating actual gains requires tracking key performance indicators such as reduced operational inefficiencies, recovered lost revenue opportunities, and fewer compliance penalties. Organizations should first identify costs of poor data quality, then calculate their investment in improvements, define relevant KPIs, and continuously monitor results.
With proper measurement, companies typically discover that reducing missing values by just 10 percentage points yields additional profit between INR 1,687,609.02 to INR 2,531,413.52 per campaign.
Conclusion
Missing data remains a critical challenge that affects organizations far beyond simple technical inconveniences. Companies lose between 15-25% of revenue due to poor data quality, while failed ML projects cost millions in wasted investments and lost opportunities.
Data quality issues demand immediate attention through systematic approaches. Organizations that implement robust data governance frameworks, automated quality checks, and comprehensive training programs see significant improvements. Success stories like the healthcare provider achieving 70% reduction in missing data demonstrate that these challenges can become opportunities for operational excellence.
The path forward requires treating data quality as a strategic priority rather than a technical problem. Companies must establish clear accountability, deploy monitoring systems, and create measurable objectives for data completeness. Those who take decisive action now position themselves to make reliable, data-driven decisions while their competitors continue struggling with incomplete datasets.
Rather than accepting data gaps as inevitable, smart organizations recognize that every missing data point represents an opportunity to strengthen their entire data infrastructure.